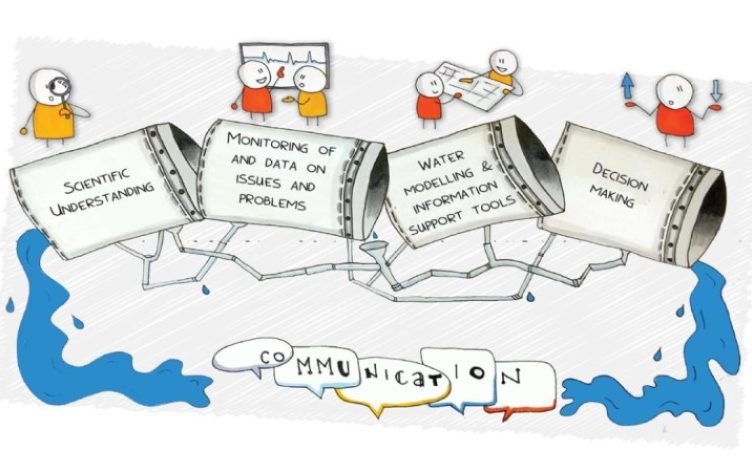
05/12/2024
Event Summary: Overview of water quality modelling projects from the Queensland Water Modelling Network with Callym Dunleavy
Read more
The adoption of linked catchment and receiving water quality models (RWQM) is relatively common in applications that look into assessing the response of a waterway to activities in the contributing catchments. A good example is the use of these models to support Healthy Land and Water’s production of estuarine health report cards in Southeast Queensland.
One of the main drawbacks in this modelling approach is the uncertainty in the calculation of pollutant load generations from the catchment models to accurately reproducing inputs into the receiving models. This uncertainty stems from structural modelling properties (i.e. time and spatial resolution) but also from assumptions to lump generation processes across vast areas with variable physical and economic (i.e. land use) characteristics. As a result, catchment models quite often lack sufficient granularity or process representation to replicate field observations. This is particularly relevant when there is a need to distinctly capture catchment response over dry and wet conditions.
With this in mind, BMT, UWA, Health Land and Water and the QWMN collaborated on a project to investigate whether machine learning techniques could be adopted to reduce the uncertainty and improve the accuracy of catchment models by incorporating specific attributes of the observations into catchment model simulation results.
Early in the process, the machine learning predictions indicated that a data driven model performed better than the incumbent catchment model, indicating that there were serious issues with the intrinsic predictive ability of the physical model. Noting that the temporal and spatial resolution of the catchment models were insufficient to replicate field observations, a model that could handle the increased resolution was required. As a result, a novel catchment model was developed to work in tandem with machine learning techniques for improved predictions.
The model was applied to the Bremer River catchment and shown to drastically improve the ability to replicate observations. PEST was used to optimize the parameter set through model regularization, while Machine Learning methods were used to improve overall model predictions. This means that the RWQMs are likely to benefit from improved catchment representation from a load generation perspective but also that research effort can be more effectively targeted to address the larger sources of uncertainty in the modelling process. It is hoped that relatively shortly this novel model structure may become commonplace to replace the existing modelling approach for more effective and accurate water quality management of Queensland waterways.
The following project team were involved in the project –
DES – Dr. Fred Bennett, Jenny Riches, Sarah Stevens and Rosie Hancock
BMT – Aditya Singh and Dr Daniel Botelho
UWA – A/Prof Matt Hipsey
HLW – Dr. Iris Tsoi
Scientific Peer Review – Dr. John Doherty (Watermark Numerical Computing)